The fog of AI and what investors are missing
Why we're still underestimating the possibilities of AI, and where we should be looking
According to some investors, we’re in the trough of disillusionment with AI. Investors who recently sung the praises of artificial intelligence now express doubts. With headlines in the WSJ like ”AI startup buzz is facing a reality check,” it is not surprising that venture capitalists are finding it hard to “dream the dream” internally.
Yet the art of investing relies on peering past the fog of today to glimpse tomorrow’s possibilities. Many of the best early stage investments were bets on markets that were just showing signs of forming. As a former investor, the question we often asked ourselves was: How big could this be if things go right?
Thesis investing requires the ability to see the present clearly, as well as the foresight to anticipate the possible steps that lead up to an amorphous—but potentially outsized—outcome. Without hard growth data, finding flaws and passing on startups is the easy task.
Take the typical venture fund model, where investors are tasked to lead only two to three investments each year. Assuming they have capacity to meet four new companies a day, this means not leaning into an investment happens 99.997% of the time. Passing is the easiest and statistically expected behavior.
What differentiates outlier investors from the pack is an understanding of one’s own epistemic confidence. Instead of seeing their assessments as binary—good investment vs bad investment—being able to recognize the degree of confidence in their conclusions and what would make them update their priors is key to a prophetic investment recommendation.
The current disillusionment seems to stem from an overconfidence in two places:
Our ability to forecast progress
Conviction that we’re looking in the right places
In Part One, I argue that investors—and even the AI experts—are bad forecasters. We have continued to underestimate the step-function changes between models.
In Part Two, I attempt to synthesize a model of where investors should be looking, both within and around AI. Most investors are looking in the wrong places.
In Part Three, I share how I’ve been thinking about where to invest, both for our own product opportunities at Anrok as well as my own portfolio. There is a lot that we don’t know, but there is more we can do to prepare.
Part One: Underestimating AI
When it comes to investing in AI, we face uncertainty on two fronts.
First, the pace of progress in AI is difficult to predict. The rapid advances in large language models (LLMs) that underpin many of the AI investing opportunities today have consistently surprised us. Second, it's challenging to imagine the impact AI could have on the economy if progress continues to accelerate. We have no lived experience with technologies that meaningfully speed up the rate of economic growth.
Overconfidence in our ability to anticipate LLM progress will lead to missed investment opportunities, both for investors as well as operators who are also internal capital allocators. By leaning on examples in our recent past, I attempt to illustrate how far our intuitions are from forecasting transformative progress and what we can do about it.
Why we’re likely overconfident in our ability to forecast AI progress
Predicting the future of LLMs is hard, especially when our familiarity with the technology is still in its infancy. The average tech worker in Silicon Valley first encountered LLMs less than one year ago. When OpenAI released ChatGPT in November 2022, they provided an interface to interact with a fine-tuned version of GPT-3.5: a follow-up to a language model that was published over two years earlier in May 2020. This upgraded 3.5 version was soon replaced by GPT-4 in March 2023.
It is no surprise that disillusionment is emerging now. No new generations of LLMs have been released since investors have habitually begun using the technology. Progress from the outside seems flat. Startups are building on models with the same capabilities to that at the beginning of the year.
However, LLMs were around prior to chat interfaces like OpenAI’s ChatGPT and Anthropic’s Claude. Without an easy way to interact with the models, it was unlikely one was exposed to the then capabilities of LLMs. Even when GPT-2 was published in 2019 - in the pre-covid era - researchers did not initially release the model weights. Few have truly experienced the capability jump between GPT-2, GPT-3, and GPT-4.
For most investors, LLMs have only become part of the everyday vernacular and workflow in the past few months. This is not an area investors can rely on intuition or experienced pattern-matching to predict progress.
To be fair, AI experts have also underestimated the performance and time-to-release of next generation LLMs. A comprehensive primer on this is Ajeya Cotra’s coverage on ML forecasting competitions and available performance benchmarks.
The short version is that superforecasters undershot reality so much so that actual capability improvements were well outside of the bell curve of consensus estimates. When AI experts were asked in mid-2022 to predict milestones like “write an essay for a high school history class,” their multi-year prediction was soon proven wrong a mere few months later.
The time between model deployments might be viewed as a trough for some. However, this lull is an opportune time to position one’s company, team, and data, to be ready to build the best products when the next generation of models arrive.
Part Two: Unlikely but possible
AI is the frontrunner to impact the pace of economic progress. Specifically, transformative AGI in the form of LLMs. Thus, LLM progress is an important variable in our future world view. An examination of how AI could impact our broader economy will reveal more about the environment that our startups will be operating in in a few years time and where the best investment opportunities may exist today.
Typically, progress comes in the form of gradual change. As the research team at Open Philanthropy puts it, most technologies don’t accelerate the pace of economic growth. They merely assist it.
The kind of progress that opens up real investment opportunities comes in the less familiar form of punctuated equilibrium. Some call these platform shifts. There is a chance that this is more than that.
The last time we experienced real change—let’s define this as the acceleration in the doubling rate of GDP—was the Industrial Revolution. This table from Bradford DeLong’s paper in 1998 examines how many years it took the global economy to double.
It took 200 years for GDP to double in the years immediately before the Industrial Revolution (1570-1765). In contrast, the doubling period immediately after took 40 years (1860-1900). This is a 5x speed up.
In the years since the Industrial Revolution, the doubling time of the economy has hovered around 20 years. This century-long trend has been so pronounced that economists have called this The Great Stagnation. Even investors who have seen multiple market cycles cannot claim to have experienced real change in the pace of progress.
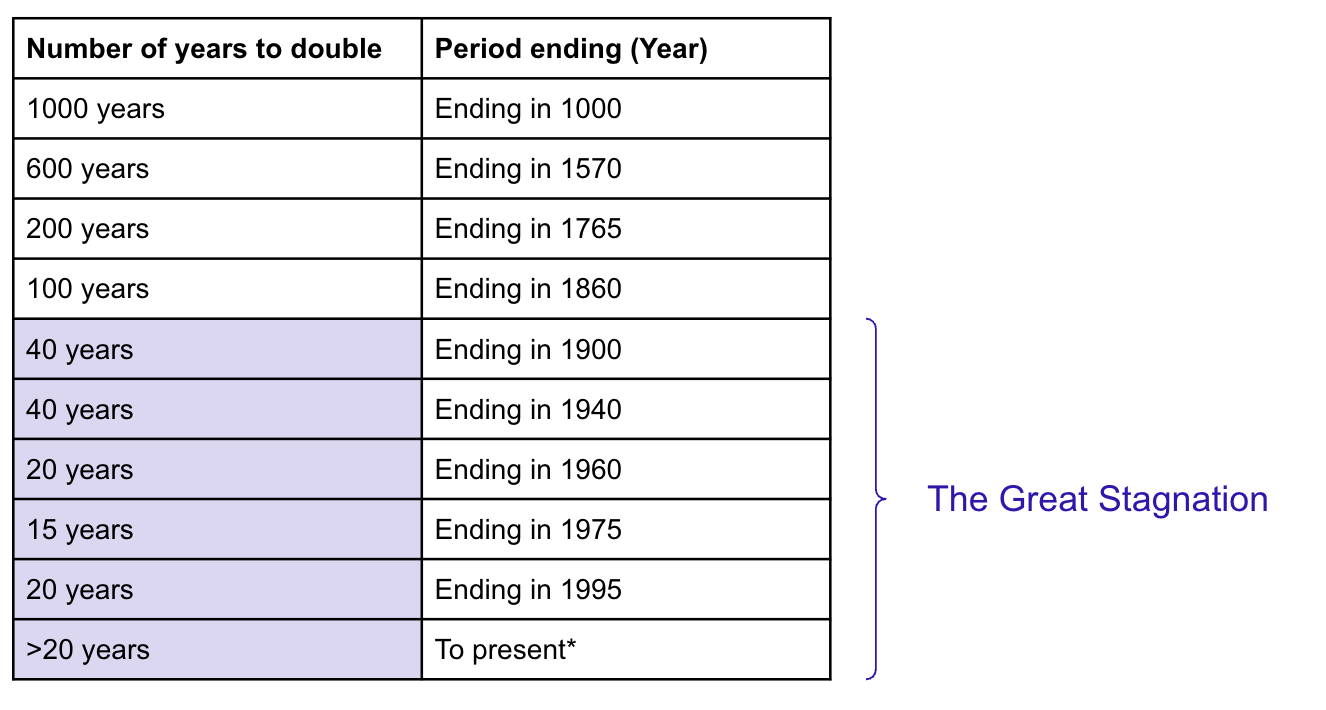
So, what does this mean for an investor’s ability to forecast progress and pick winners?
Today’s investor has been placing bets in a static world. While technology has led to economic growth, it has not meaningfully accelerated the rate of growth in recent years.
Given this historical backdrop, how confident are you that progress in the next hundred years looks similar to the last hundred? Even if you’re skeptical of AI, a longer view of history suggests that the status quo is not the only potential reality.
If we continue to underestimate the step-function changes between models and take a narrow view of technological progress, our ability to pick the downstream (or upstream) winners looks more like a gamble than a calculated bet.
Part Three: Unexpected winners
Today’s environment for early stage investing is unlike any other. Whether you believe this is simply a long awaited platform shift or glimpse possibilities of more transformative progress, there has never been a more exciting time to invest in and build technology.
We’re being shortsighted with software TAM
Recent developments in AI are already accelerating software development productivity. GitHub’s CoPilot claims to increase developer output by 1.5x with just the models we have today. Besides expansion of the existing software market, AI will further digitize analog industries like customer support, consulting services, tutoring, art, and much more.
The impact of digitizing the analog is underrated. Many investor forecasts of TAM miss the mark on how big and how fast this shift is going to be. The TAM calculations I’ve seen from investors take existing AI revenue data (i.e. looking at sector productivity gains of current models) instead of working from looking at how large existing analog industries are.
The problem of relying on AI revenue today is that it has a short history and only considers capabilities of the current models (recall the step function changes in Part One). Despite reports that AI revenue generated by these players today are impressive and massive—The Information has reported that OpenAI is generating $1B in annual revenue—investors seem to forget this revenue was essentially zero a year ago.
To illustrate how big the outer circle of this could be, the consulting services market is about US$300B today alone (Statista, 2023). How much of that industry will be transformed by and perhaps even enlarged by the next-generation model?
The growth potential of these industries when coupled with AI is unprecedented. Investors still don't seem to be factoring in the speed at which revenue has scaled, perhaps dismissing them as outliers. In the short few months, there are product examples across different domains, such as AI chatbot, AI art, AI programming and more that have scaled from zero to hundreds of millions in revenue. Factoring speed into TAM projections produce some weird results that could make some investors uncomfortable.
Where to look
If I were to distill what is interesting about the Silicon Valley startup investing environment today into five general buckets, they would be:
Research-driven teams that have many years of machine learning experience, like Ilya Sutskever at OpenAI, Dario Amodei at Anthropic, Noam Shazeer at Character, and David Holz at Midjourney.
These tend to be capital intensive bets that have high valuations out of the gate, which are hard for smaller funds to stomach. Here, you’re betting on the caliber of technical talent early and their ability to attract other top notch researchers. The good news for investors is that the best teams have a long track record of leading and publishing conference winning papers, a proxy for whether they can stay on the cutting edge.Growth stage startups with a corpus of valuable data and a team that can innovate, like Jason Boehmig at Ironclad, Tim Zheng at Apollo, and Mathilde Collin at Front.
LLMs are primarily good at two things: content creation and data synthesis. Arguably the latter is the precursor step to unlocking the most value. Even if your product is ultimately creating content, outputs based on some personalization and understanding of the user’s context tend to be more useful. It all starts with having proprietary industry or user specific data.Companies who have yet to ship a public LLM-powered product could still be an AI gem. The best product teams know that any products shipped to users today will require ongoing maintenance. While they might iterate quickly behind the scenes, they ship intentionally. God forbid they ship a simple chat interface product named InsertCompanyNameGPT…
In addition, the capabilities of LLMs are prone to punctuated equilibrium (see Part One). Companies might need the next generation model to make their ideas possible. Hallucinations and the limited number of parameters might mean today’s models aren’t smart enough for the tasks at hand. Those who have access to the right data and sit in a strategic place in a user’s workflow are best positioned to win when the time comes.
Startups that were “AI” from the get-go, such as Glean by Arvind Jain, Forethought by Deon Nicholas, Moonhub by Nancy Xu, and Harvey by Gabriel Pereyra.
This is the category that is getting the most investor attention. Many exciting applications have yet to be built around LLMs. There is room to build products around specific workflows and deliver a superior user experience.
Similar to #2 of companies, access to or aggregation of proprietary data is paramount to building a defensible business. Without a data strategy, many startups will never get out of being a thin wrapper around an LLM.
The obvious picks and shovels on the path to transformative AI, like Scale by Alex Wang, Tonic by Ian Coe, Gantry by Josh Tobin, and Coactive by Cody Coleman.
Infrastructure for model training, data, or deployment are classic derivative bets on AI. Opportunities range from chip hardware to model deployment platforms. Companies like Scale were ahead of the curve and outexecuted competition to lead the data labeling space. Other companies like Tonic AI take the synthetic approach to meet the demand for data. Identifying which picks and shovels deliver real and enduring value, coupled with the team that has the horsepower to execute, is a tried and true investment strategy.
The unexpected picks and shovels on the path to transformative AI, see the approaches below.
This is the category most overlooked. As LLMs get smarter, every engineer can be transformed into a 10x contributor. Without development resource constraints, every startup can be a compound startup. Software will truly “eat the world”.
Companies facilitating digital commerce are the hidden backbone of the rise of AI. It’s another approach to the picks and shovels / AI Index bet. Here is how I think about the different categories as of September 2023:
❓Create software
I’m still not convinced that there is much within the “Create software” category.
Coding productivity does feel like something the AI assistants built by OpenAI and Anthropic will do well. Given how closely Microsoft / Github Copilot is with OpenAI and was built by the team at OpenAI, I group them largely in the same bucket. Maybe Replit has the best chance to do this well, but I haven’t looked into the company in detail since 2017.
Building / connecting different APIs also seem like something that can be easily disrupted by the next-generation of custom programming assistants. That said, if you’re unifying regulated private APIs that you don’t have access to as the user, like Plaid, Modern Treasury, or Column, I still think there is a moat here.
✅ Manage, deploy, and secure software
✅ Deliver software
End-user access controls and / or auth software like Multiparty AI from Indent by Fouad Matin
Procurement software that enables companies to organize and effectively purchase more software like Zip by Rujul Zaparde
Compliance software that enables companies to sell software across borders like Anrok (where I’m all-in)
Above reflects my personal opinion on the categories that are interesting and is not intended as investment advice 🙂
It is easy to be overconfident in early stage investing where the universe of companies is large and you’re rewarded to be decisive. Most of the time, it pays off to move quickly. Identifying when a key input to our world view has changed provides an opportunity to step back, reassess priors, and recalibrate.
This three-part post has attempted to (0) address some of the herd mentality and disillusionment by (1) illustrating how we’re underestimating AI progress, (2) outlining how we’re thinking too narrowly about unlikely but possible futures, and (3) providing a framework on where to look for the unexpected winners in this AI environment.
At Anrok, we believe the Internet economy is only just beginning. We’re at our Wright brothers moment, where the plane looks nothing like where we might go in a short few years time. If you’re building something ambitious in the software space and could use help expanding across state and global borders, I’d love to connect. ⊞